last modified: 2022-12-16
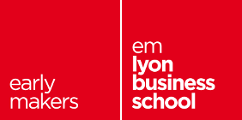
Not a closed list, not a recipe! Rather, these are essential building blocks for a strategy of value creation based on data. |
1. Predict
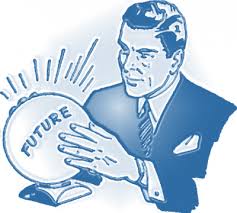
a. Examples of companies
-
Predicting crime
-
Predicting deals
-
Predictive maintenance
b. Obstacles and difficulties
-
Risk missing the long tail, algorithmic discrimination, stereotyping
-
Neglect of novelty
2. Suggest
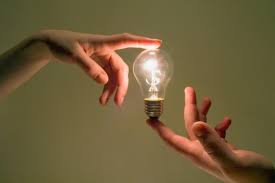
a. Examples of companies
-
Amazon’s product recommendation system
-
Google’s “Related searches…”
-
Retailer’s personalized recommendations
b. Obstacles and difficulties
-
The cold start problem, managing serendipity and filter bubble effects.
-
Finding the value proposition which goes beyond the simple “you purchased this, you’ll like that”
3. Curate
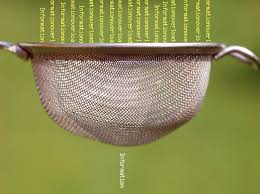
a. Examples of companies
-
Clarivate Analytics curating metadata from scientific publishing
-
Nielsen and IRI curating and selling retail data
-
ImDB curating and selling movie data
-
NomadList providing practical info on global cities for nomad workers
b. Obstacles and difficulties
-
Slow progress: curation needs human labor to insure high accuracy, it does not scale the way a computerized process would.
-
Must maintain continuity: missing a single year or month hurts the value of the overall dataset.
-
Scaling up / right incentives for the workforce: the workforce doing the digital labor of curation should be paid fairly, which is not the case yet.
-
Quality control
4. Enrich
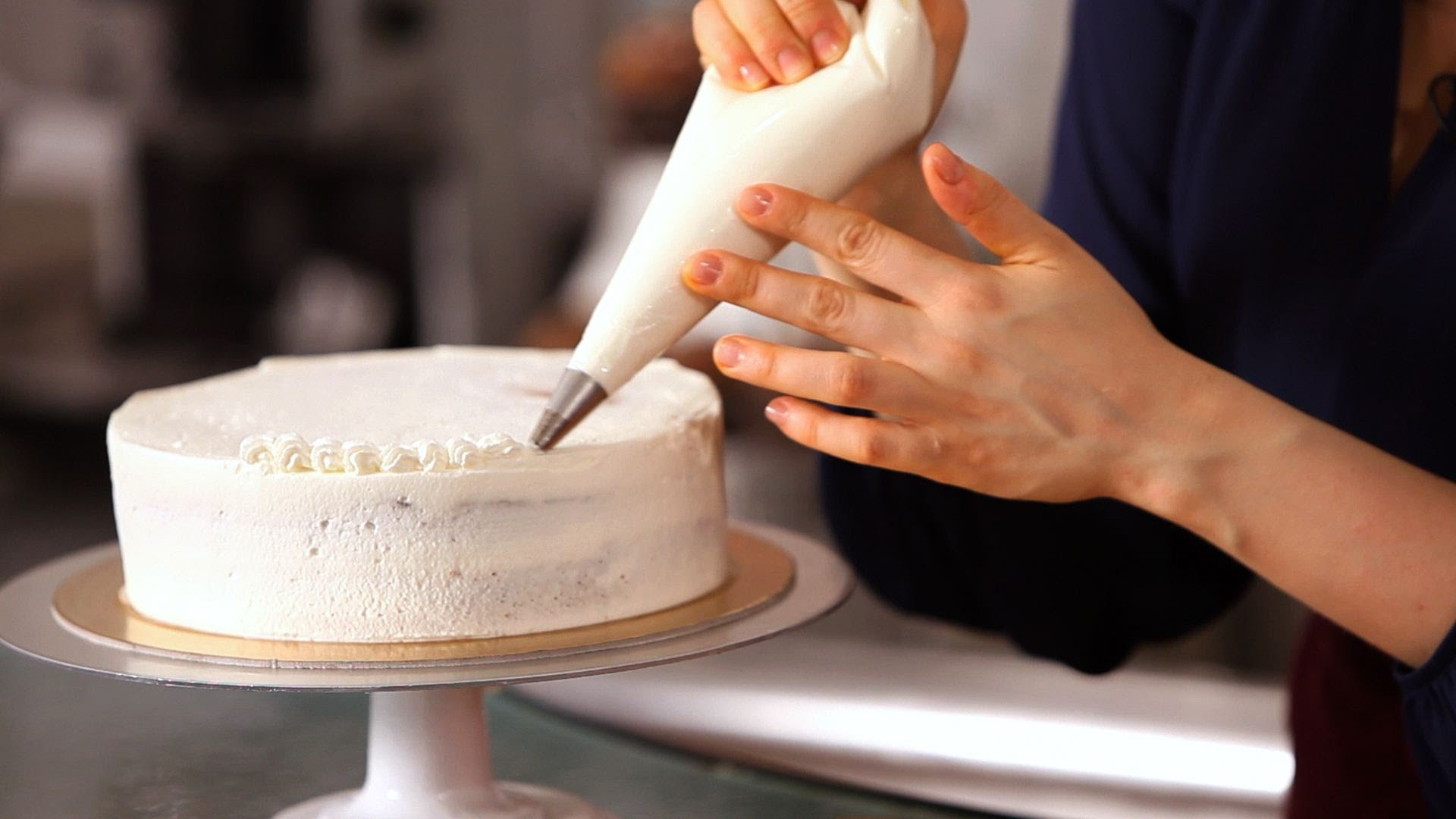
Examples of companies
-
Selling methods and tools to enrich datasets
-
Selling aggregated indicators
-
Selling credit scores
Obstacles and difficulties
-
Knowing which cocktail of data is valued by the market
-
Limit duplicability
-
Establish legitimacy
5. Rank / match / compare
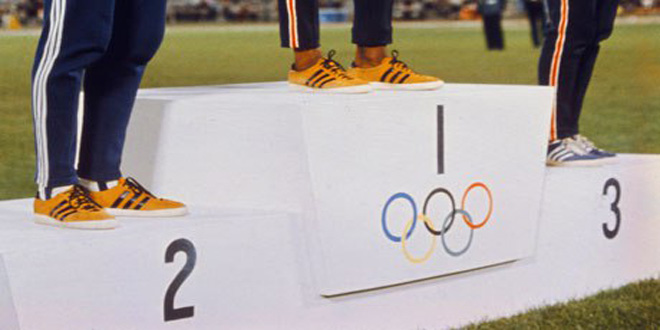
Examples of companies
-
Search engines ranking results
-
Yelp, Tripadvisor, etc… which rank places
-
Any system that needs to filter out best quality entities among a crowd of candidates
Obstacles and difficulties
-
Finding emergent, implicit attributes (imagine: if you rank things based on just one public feature: not interesting nor valuable)
-
Insuring consistency of the ranking (many rankings are less straightforward than they appear)
-
Avoid gaming of the system by the users (for instance, companies try to play Google’s ranking of search results at their advantage)
6. Segment / classify
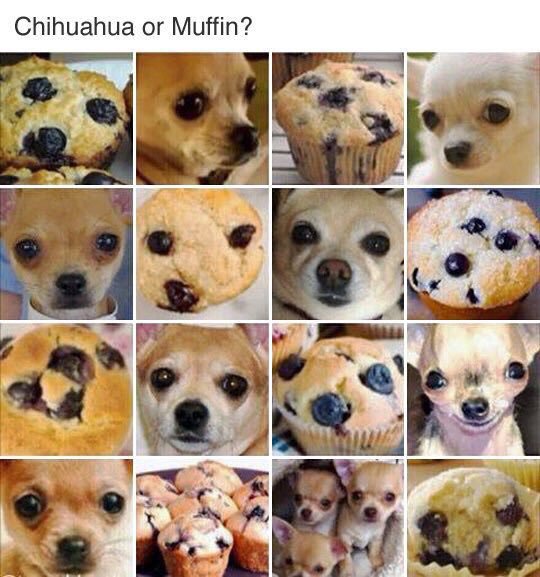
Examples of companies
-
Tools for discovery / exploratory analysis by segmentation
-
Diagnostic tools (spam or not? buy, hold or sell? healthy or not?)
Obstacles and difficulties
-
Evaluating the quality of the comparison
-
Dealing with boundary cases
-
Choosing between a pre-determined number of segments (like in the k-means) or letting the number of segments emerge
7. Generate / synthesize (experimental!)
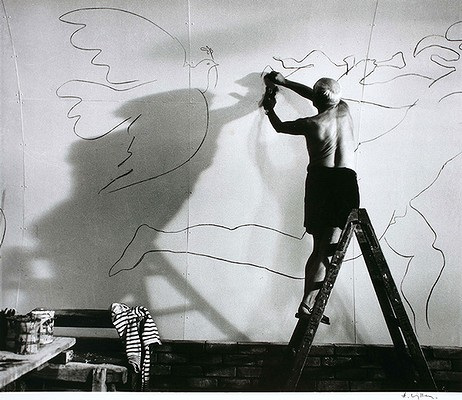
Examples of companies
-
Intelligent BI with Aiden
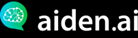
-
wit.ai, the chatbot by FB
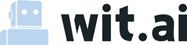
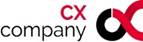
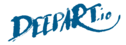
-
Close-to-real-life speech synthesis

-
Generating realistic car models from a few parameters by Autodesk
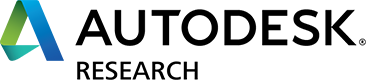
-
Generating summaries and comments from financial reports Yseop
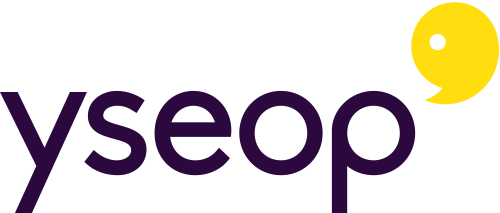
A video on the generation of car models by Autodesk:
Obstacles and difficulties
-
Should not create a failed product / false expectations
-
Both classic (think of
) and frontier science: not sure where it’s going
Combos
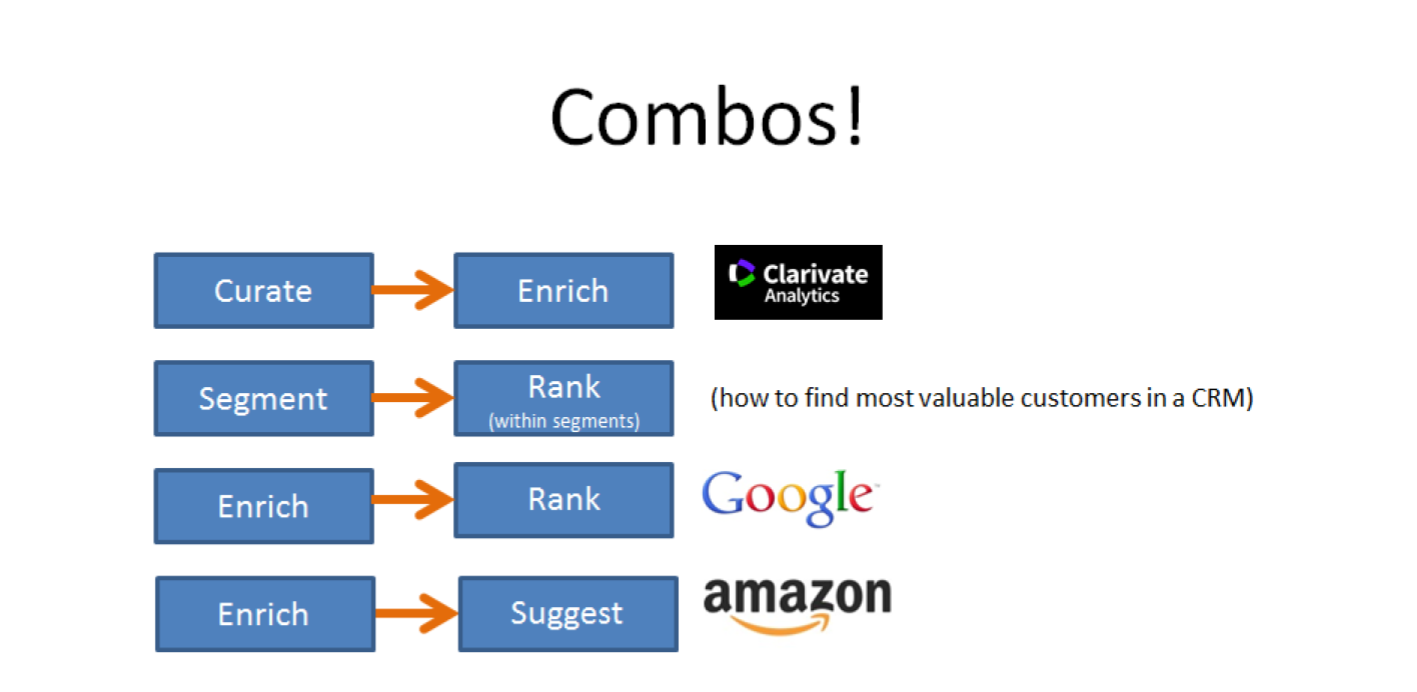
The end
Find references for this lesson, and other lessons, here.
This course is made by Clement Levallois.
Discover my other courses in data / tech for business: https://www.clementlevallois.net
Or get in touch via Twitter: @seinecle r business: https://www.clementlevallois.net
Or get in touch via Twitter: @seinecle r business: https://www.clementlevallois.net
Or get in touch via Twitter: @seinecle r business: https://www.clementlevallois.net
Or get in touch via Twitter: @seinecle @seinecle get in touch via Twitter: @seinecle get in touch via Twitter: @seinecle get in touch via Twitter: @seinecle get in touch via Twitter: @seinecle get in touch via Twitter: @seinecle get in touch via Twitter: @seinecle get in touch via Twitter: @seinecle get in touch via Twitter: @seinecle get in touch via Twitter: @seinecle